Customer-GPT model: Infrastructure, Language Model Selection, Data Sovereignty and Outlook
- mhaupt5
- Oct 11, 2023
- 2 min read
Updated: Jan 11, 2024
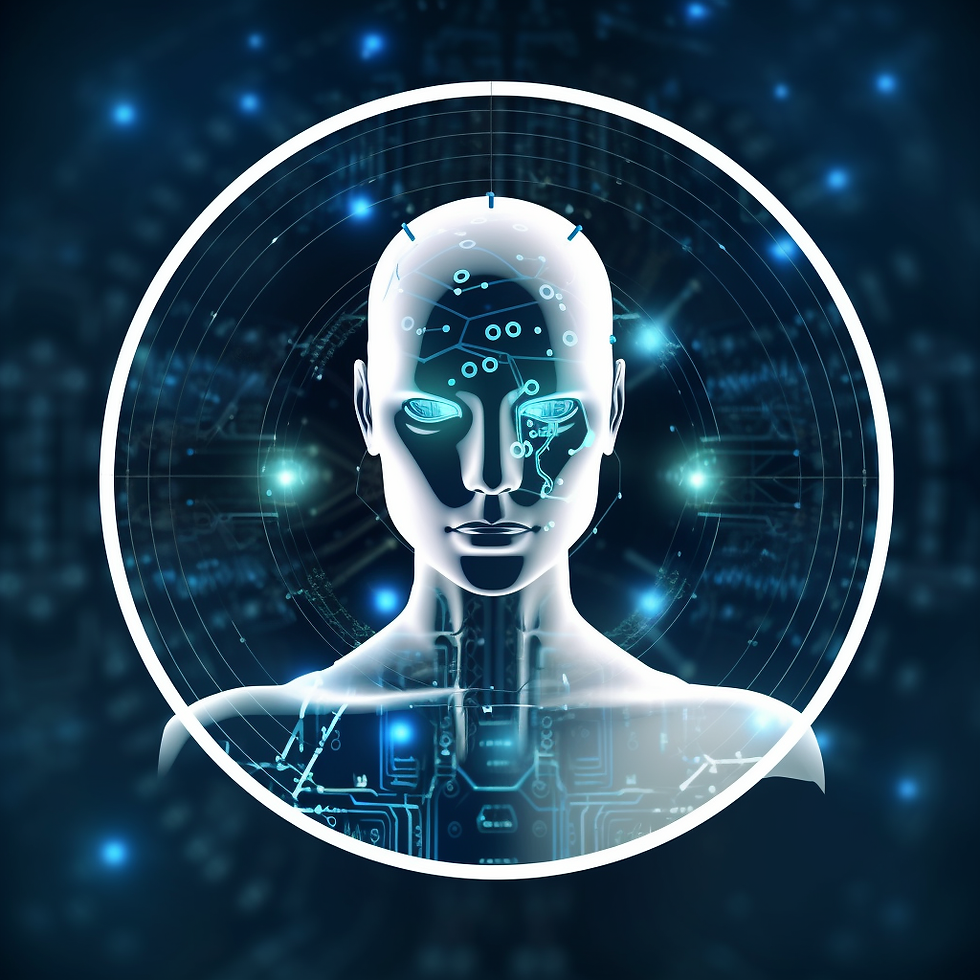
Introduction
The implementation of Generative Pre-trained Transformers (GPT) for customer service, engagement, and experience is an area of active research and development. As more companies look to automate and optimize their customer interactions, the choice of infrastructure, the selection of Language Lifecycle Management (LLM), and the crucial aspect of data sovereignty come to the fore. In this blog post, we'll delve into these components to provide a comprehensive guide for anyone considering GPT for customer-related applications.
Private transformer-type chat model, using specific corporate data sets to create its knowledge base. Delivering data privacy and security - safe for client to upload company-internal data.
Why?
Modern and powerful AI solutions exists, many of which share one significant disadvantage: enterprise-users give up sovereignty over their internal data. The Private Customer-GPT features an enterprise-ready AI solution that offers user management, data sovereignty, cost efficiency, and which answers purely based on specific uploaded company information.
Some basic infrastructure needs to consider
There are multiple infrastructure needs to consider. Here are a few examples: Scalable Computing & Data Storage Resources like:
GPT models can be resource intensive. Therefore, a scalable cloud or on-premises solution is advisable for handling peak loads.
High-speed, secure data storage is a must for storing conversational data and model parameters.
Low-latency, high-throughput networking solutions ensure smooth data flow between the model and the customer interaction platforms.
Moreover, it is helpful to implement monitoring and management tools. It is essential for overseeing system health, performance, and security.
Language Lifecycle Management (LLM) to verify
What kind of model you need depends on processes and your workflows. Some examples to check are small scale vs. large scale domain-specific models as well as Multi-Lingual Requirements. Costs of the models and resource consumption are getting critical elements as well.
Data Sovereignty and Compliance to reflect
To comply with data sovereignty laws, ensure that the data centers you use for storage are located in the appropriate jurisdictions. Data should be encrypted both in transit and at rest. Employ robust authentication mechanisms to ensure data integrity. Implement data governance policies to track who has access to data, how it is used, and where it is stored. Ensure your infrastructure provider complies with GDPR, CCPA, or any other relevant data protection regulations.
The Road Ahead
While GPT models offer revolutionary capabilities for automating and enhancing customer as well as employee interactions, their implementation is not without challenges. Multiple aspects like costs, data management, interoperability or infrastructure topics needs to be defined. Adopting GPT for customer interactions necessitates a multi-faceted approach that involves infrastructure planning, LLM selection, and a keen focus on data sovereignty. As the technology matures, organizations that successfully navigate these complexities stand to gain a competitive edge in delivering an unparalleled customer experience.

Comentarios